In recent years, the energy sector has witnessed a transformative shift driven by the rapid advancement of machine learning (ML) technologies. This article explores the cutting-edge applications of machine learning for energy production, distribution, and consumption. By leveraging the power of data-driven insights and predictive modeling, ML is poised to unlock unprecedented efficiency, sustainability, and innovation within the energy industry.
The energy landscape is undergoing a profound transformation as the world grapples with the challenges of climate change, resource depletion, and ever-increasing energy demands. Traditional energy systems are being disrupted by the emergence of renewable sources, decentralized generation, and smart grid technologies. In this dynamic environment, machine learning has emerged as a powerful tool to optimize energy systems, enhance decision-making processes, and drive innovation.
Machine Learning Applications in Energy
Renewable Energy Machine Learning based Forecasting and Integration
Machine learning algorithms are revolutionizing the forecasting and integration of renewable energy sources, such as wind and solar energy prediction using machine learning. By analyzing historical data and real-time meteorological conditions, ML models can accurately predict energy generation, enabling better resource planning and grid integration. This improved forecasting capability enhances the reliability and efficiency of renewable energy systems, facilitating their widespread adoption.
Energy Demand Prediction and Load Forecasting
Understanding energy demand patterns is crucial for efficient energy management and resource allocation. ML techniques can analyze vast amounts of data, including weather conditions, historical consumption patterns, and demographic factors, to predict energy demand with high accuracy. Machine learning for power systems aids in optimizing power, reducing energy waste, and implementing demand-response programs, ultimately leading to cost savings and improved grid stability.
Predictive Maintenance and Asset Management
Energy infrastructure, such as power systems, transmission lines, and distribution networks, requires regular maintenance and monitoring to ensure reliable operation. ML algorithms can analyze sensor data, asset performance metrics, and historical maintenance records to predict potential failures and recommend preemptive maintenance strategies. This predictive maintenance approach minimizes downtime, extends asset lifespan, and reduces operational costs.
Energy Efficiency and Building Management
ML techniques can be applied to optimize energy consumption in residential, commercial, and industrial buildings. By analyzing data from sensors, occupancy patterns, and building characteristics, ML models can identify energy-saving opportunities and automatically adjust heating, ventilation, air conditioning, and lighting systems. This approach results in significant energy savings, reduced carbon emissions, and improved occupant comfort.
Smart Grid and Microgrid Management
The integration of ML into smart grid and microgrid systems enables intelligent energy management through machine learning. ML algorithms can analyze real-time data from distributed energy resources, energy storage systems, and consumer demand patterns to optimize energy flows, reduce losses, and enhance grid resilience. This intelligent management approach facilitates the seamless integration of renewable energy sources and enables effective demand-side management.
Challenges and Considerations
While the applications of ML and artificial intelligence (AI) in the energy sector are promising, there are several challenges and considerations that need to be addressed:
Data Quality and Availability: ML models rely heavily on the quality and availability of data. Ensuring accurate and comprehensive data collection from various sources is crucial for successful ML implementation.
Model Interpretability and Transparency: Some ML models, such as deep neural networks, can be opaque and difficult to interpret, posing challenges in understanding and explaining their decision-making processes. Efforts should be made to develop interpretable and transparent ML models for critical energy applications.
Cybersecurity and Privacy: As energy systems become increasingly digitized and interconnected, cybersecurity risks and data privacy concerns arise. Robust security measures and data protection protocols must be implemented to safeguard energy infrastructure and sensitive consumer data.
Regulatory and Policy Considerations: The adoption of AI and ML technologies in the energy sector may require updates to existing regulations and policies. Collaboration between industry stakeholders, policymakers, and regulatory bodies is essential to create a supportive and enabling environment for ML-driven innovations.
As an expert market intelligence firm with tons of experience in machine learning (ML) applications in the energy sector, we provide valuable insights and services to many of our energy sector clients who are interested in exploring the possibilities of Machine Learning for their business. Here are some key topics which are of interest to many of our energy sector clients:
- Collaborate with industry experts, researchers, and thought leaders to gain deeper insights and stay ahead of emerging trends.
- Assess the adoption rates, growth potential, and market size for various ML applications in different energy domains (renewable energy, oil and gas, utilities, etc.).
- Identify emerging technologies, disruptive innovations, and potential game-changers in the ML-energy space.
- In-depth research and analysis of the current and future market trends, regulatory changes, and challenges related to ML applications in the energy sector.
- Analyze the competitive landscape, identifying key players, their offerings, and market positioning.
- We can help you with due diligence for strategic partnerships with leading energy companies, ML technology providers, research institutions, and industry associations.
- Leverage advanced data analytics techniques to derive actionable insights from complex energy and ML-related data sets.
- Automized data collection from multiple sources, model development, and deployment recommendations for ML applications in the energy sector.
- Develop interactive data visualization tools and dashboards to present market intelligence in a clear and compelling manner.
- Data-driven decision support services to energy companies, enabling them to make informed strategic decisions based on robust market intelligence.
Case Study: Data-Driven Insights for Renewable Energy Integration
Background: Our client, a leading utility company, sought to optimize their renewable energy portfolio and enhance grid stability through data-driven insights.
Challenge: The client faced challenges with large volumes of diverse data, complexity in analyzing data from disparate sources, difficulty in forecasting renewable energy generation, and limited insights into optimal energy mix and resource allocation.
Our Approach:
- Data ingestion and preprocessing from various sources, including weather data, energy generation data, and grid performance data.
- Feature engineering and dimensionality reduction techniques to extract relevant features and reduce data complexity.
- Predictive modeling and forecasting using machine learning models, including deep neural networks and ensemble techniques, to accurately forecast renewable energy generation.
- Data visualization and reporting through interactive dashboards and comprehensive reports.
Results and Impact:
- Improved renewable energy forecasting accuracy of over 90%.
- Optimized energy mix and resource allocation, resulting in a 15% reduction in operational costs and a 20% improvement in grid stability.
- Enhanced decision-making through data-driven insights and interactive dashboards.
- Increased sustainability and reduced carbon footprint by 18% through optimized renewable energy integration.
This case study showcases our ability to leverage advanced data analytics techniques to derive actionable insights from complex energy and ML-related data sets, enabling clients to achieve operational excellence, cost savings, and sustainability goals.
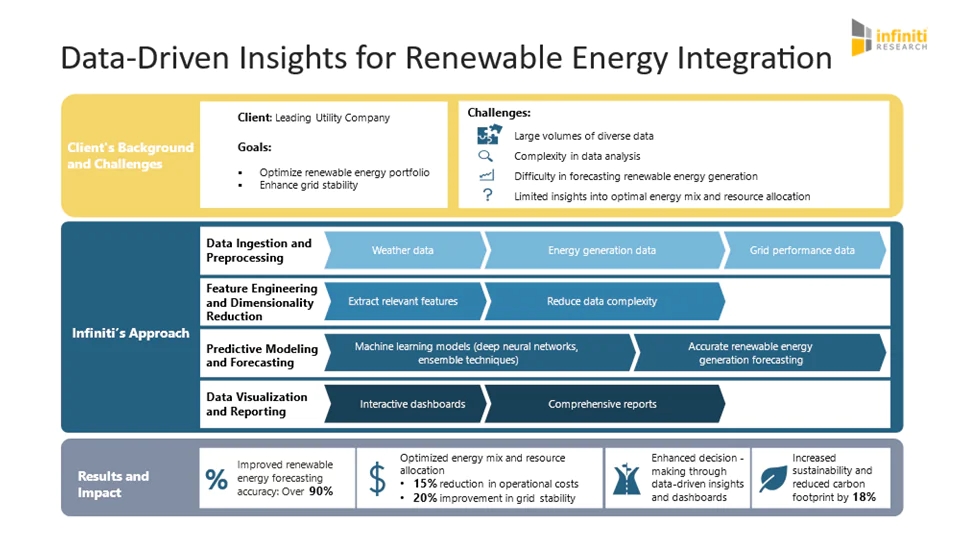
Conclusion:
In conclusion, machine learning presents transformative opportunities for the energy sector to address climate change, optimize resource usage, and drive sustainability. By leveraging data-driven insights and predictive modeling, ML techniques can revolutionize energy production, distribution, and consumption. However, successful implementation requires overcoming challenges around data quality, model transparency, cybersecurity, and regulatory hurdles. Collaboration across stakeholders is key to fostering an environment conducive to responsible ML adoption. As the energy landscape evolves, embracing ML will be instrumental in achieving a more efficient, resilient, and sustainable energy future. The time to harness ML’s potential is now for those seeking a competitive edge in the rapidly transforming energy market.